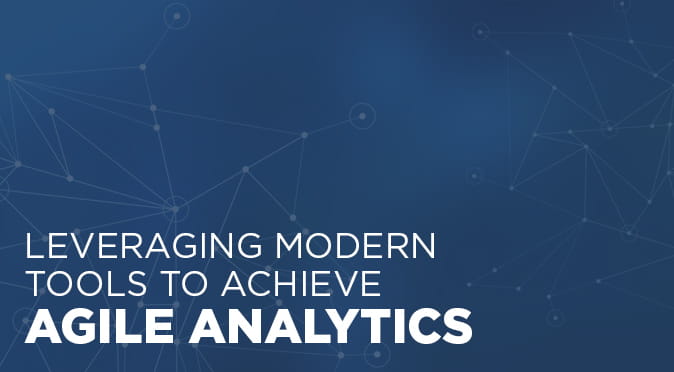
Organizations have grown increasingly enthusiastic about the business insights and other benefits that big data analytics can deliver, and rightfully so. But as they pursue the promise of business analytics, companies often come face to face with some hard truths. One of the most problematic: following the traditional process of first creating a data warehouse and then analyzing the data within it can be costly and time consuming. By the time organizations have the results in hand, they may no longer be relevant to current business demands.
Modern analytics tools can help solve this problem by supporting “agile analytics,” both to perform rapid proof of concept and to speed the build-out of more comprehensive infrastructure. In this blog I answers a few of the most common questions associated with agile analytics.
Instead of starting with data warehouses, which can be multi-year projects, companies can use modern tools such as Microsoft’s Power BI to take the data they already have and quickly mock up useful reports. The data might not be as cleansed and formatted as if you had a data warehouse, but business users can see value and you can generate excitement early on. You can get buy-in quickly because customers are demanding results faster than ever before.
Once you’ve confirmed the value of the insights provided, you can start building the plumbing behind the reports to fully operationalize and automate them. In essence, you’re giving the business units a reason to invest before undertaking more comprehensive and costly analytics projects.
Definitely. When business users see they can get better insights through data analytics, they become invested in the process. Their early involvement can then help data engineers more efficiently perform governance tasks such as determining which data sources have the most business value, which fields to include in reports, and so forth.
It’s common for data scientists to spend 80% of their time performing ETL (extract, transform, and load) tasks before the data can even be analyzed. By helping to ensure that only the most relevant data sets go through this process, business users can greatly reduce these time-consuming data preparation processes.
Agile analytics helps business people see the art of the possible. That’s an important first step in helping to identify advanced analytical opportunities. So many business people don’t even understand the data they already have today. Once they have a better understanding they are better able to work with data scientists to capitalize on machine learning (ML) opportunities.
We worked on an agile analytics approach with one mid-market company, doing lots of experimenting and demonstrating. Through that process, we decided to create a machine learning algorithm so that the system would automatically recommend new products to renewing customers based on what similar customers had purchased. That company is now seeing $20 million in additional revenue annually thanks to the machine learning solution that the agile analytics used.