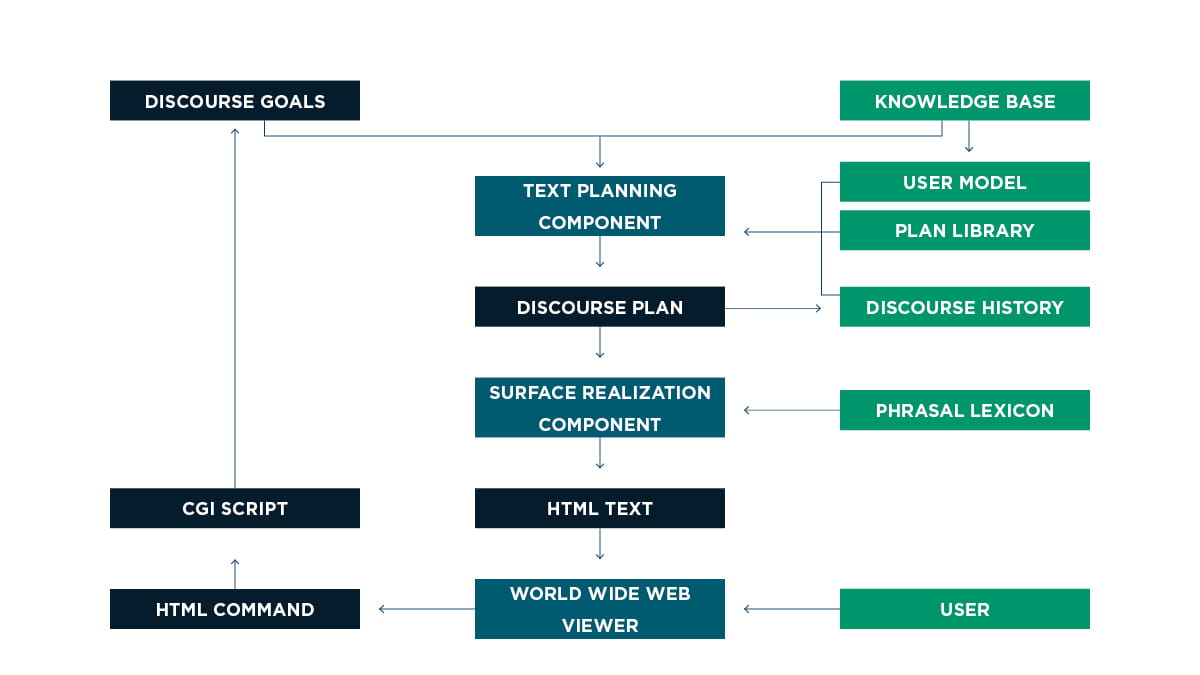
Natural Language Generation (NLG) is a form of artificial intelligence (AI) which takes an input of data and produces a narrative similar to how a human would interpret the data. NLG technology can be very transformative when used in business situations, especially in industries that are heavily dependent on generating text summaries based on straightforward conclusions drawn from structured data and that lack the capital to support a large workforce.
The purpose of this article is to provide a brief background on natural language generation technology, analyze industry scenarios and address the considerations for selecting and implementing NLG applications. We’ll also uncover how organizations in the energy & utilities, life sciences, and financial services industries can focus on business intelligence (BI), improve operations—and, more importantly, take the right steps toward improving their management insight through the use of natural language generation.
The narratives generated by natural language generation systems use both machine learning algorithms and pre-set parameters to express data inputs as they would be written naturally, by a real person. While natural language generation has recently become a popular tool across certain industries, Natural Language Processing (NLP), the broader field to which NLG belongs, has long been researched by computer scientists. This technology has been a topic of research since the 1950s when computer scientists began thinking about the future of computing. Since then, each wave of AI research has brought more functional, human-sounding NLG systems.
Generally, NLG is performed in two different phases: the strategic phase (what to say) and the tactical phase (how to say it). Using statistical analysis, a NLG program is able to predict and mimic the human voice in the tactical phase.
Early NLG platforms were developed for purely academic and research purposes. Historically these systems were based on a knowledge base that was hand constructed from encyclopedia articles and various taxonomic classes. The following excerpt is an example of an early NLG generated narrative, using the late 1990s developed PEBA-II system:
“The Echidna is about the same length as a domestic cat. It ranges from 2 kg to 7 kg in weight. It has a browny black coat and paler- coloured spines. It has a small head. It has a prolonged, slender snout. It has no teeth. It uses its extensible, sticky tongue for catching ants, termites and other small insects.”
The narrative generation process used in PEBA-II is detailed in the flow chart:
In the past decade, companies dedicated to providing NLG services in the B2B market have been steadily growing. In this commercial space, the largest use of NLG is in a data-to-narrative manner; combining data analytics into the strategic phase of NLG. Currently, only structured data is compatible with these NLG commercial technologies. Structured data is easily searchable by these algorithms because it has searchable fields and associations, while unstructured data does not. This combination has already disrupted many industries including financial services, e-commerce, and even compliance.
The current vendor landscape for NLG consists of mostly small, equity funded companies head-quartered both nationally and internationally. The market for NLP was $5.7B in 2015 and is estimated to grow to $13.4B in 2020, according to a market analysis completed by Markets and Markets. In the following sections, we will highlight the benefits and considerations of implementing NLG technology through different industry use cases.
Generating natural language plays an important role in the financial services industry. Used by financial advisors, as- set managers, and portfolio managers alike, NLG technology helps create personalized portfolio updates for investors. These reports can then be used to either support decision making by portfolio managers, or to share with investors as an update on their investment portfolio. The commentaries normally detail things such as the performance of the portfolio and market trends. The narratives generated provide insights similar to those a dedicated business analyst might provide.
In a case study by one Narrative Analytics technology company, financial asset management clients and asset managers were able to use NLG technology to turn attribution data files into fact sheets with both commentary and graphics. The software was able to produce content in various languages, and file types for faster distribution of information across multiple geographies.
In the insurance industry, NLG plays a big role not only in generating claims reports and performance reports, but also in supporting call center employees by providing real-time feedback. By using customer survey data, NLG systems are able to provide insights on the pain points for individual call center employees. This real-time feedback allows employees to focus on improving specific aspects of the customer service experience which, in turn, increases customer satisfaction.
Below is an excerpt of an NLG-generated call center employee feedback report using Narrative Science’s platform:
“With an 88% customer satisfaction rating, you have demonstrated solid service and commitment to our customers, though you still have room to strive for excellence. Working on executing your disconnect rate and speak to other representatives to find ways to improve performance.
Congratulations on your continued success regarding call resolution performance. Keep up the good work on closing out your tickets.”
For energy & utility companies, NLG can be a powerful force in providing usage summaries for customers, detailing how their utility usage has changed over a period of time. This can be important to support an overall effort to conserve resources, particularly in geographic areas like California, which has been in a drought for over four years. By generating relevant and frequent reports, utility companies can engage their customer base and encourage environmentally conscious habits. This may eventually lead to increased capacity that the utility could provide to its customer base, ultimately increasing the company’s bottom line.
For the healthcare & life sciences industry, companies are just starting to understand potential benefits of generating natural language. Initial opportunities that present themselves are in the areas of being able to summarize results without bias, evaluate large data sets quickly, transform those data sets into knowledge, and facilitate personalization of data. The FDA has not yet published guidance or abstracts on the use of NLG (or NLP). Companies should consider assessing use with validated or patient information.
One example use case could be in medical affairs, where pharma companies have stored thousands of publications and library responses to medical inquires by patients and healthcare providers.
NLG can help call center and medical center providers process these requests and find the most appropriate response to specific requests based on the myriad of possible responses based on country specific regulatory requirements and therapeutic areas.
One of the more unique uses of natural language generation in industry is its role in the compliance space. As an algorithm- driven tool, there is inherently a lack of human- bias in the narratives generated. From a compliance perspective, the benefits of using this tool directly impacts the level of assurance. NLG has been implemented to support the identification of money-laundering activities within companies. This is done by analyzing company data and providing “Suspicious Activity Reports” based on anomalous behaviors evident in the records. The narratives are updated in real-time based on changes in data, and are able to maintain a consistent format for reports all throughout a firm.
As shown through the aforementioned industry scenario cases, natural language generation platforms can be very transformative when used in business situations, especially in industries that are heavily dependent on generating text summaries based on straightforward conclusions drawn from structured data and that lack the capital to support a large workforce. NLG implementations can also open doors for companies to expand beyond their current capacities by allowing employees to focus on tasks that are less back office in nature. Additionally, a single portal subscription annually varies by vendor but could be as low as $16,000 which is much more cost effective than 2015’s average American income of $53,657 as determined by the U.S. Census Bureau.
Other benefits to highlight are the dynamic and impartial nature of natural language generation technology. In most businesses, relevant data and information can change on an hourly basis. These businesses benefit greatly from NLG technology’s real-time data analytics capabilities. The narratives update in real-time to changes made in the data, making it a dynamic tool that can keep up with financial markets and other information driven industries.
NLG technology is by no means the be-all end-all solution for data analytics and narrative generation. In order to reap the benefits of NLG technology, there are certain important considerations that need to be kept in mind.
The first and the foremost consideration for NLG is the selection of the appropriate application. Although the industry scenarios mentioned above show a wide breadth of applications, the key points to consider when choosing an NLG technology are business criticality and the scope of the generated narrative. For example, in a financial advisory domain NLG is often restricted to provide narratives around what happened and why, whereas the actual financial advice is provided by the advisor.
Another consideration is that NLG market solutions can currently only digest clean, structured and centralized data. This means that NLG works best in companies that already use a sound data management strategy. On the other hand, the implications are that some companies may need to first invest in a data management solution before considering the implementation of natural language generation.
NLG evaluation techniques play a crucial role in selecting the most appropriate system.
Both these frameworks are not feasible independently due to the amount of effort and investment required to either put together a panel to go through a significantly large number of narratives or preparing multiple narratives for the same dataset to feed into the machine learning frameworks.
In our opinion the ideal approach would be to combine the above mentioned industry frameworks for an optimal solution. By combining both the frameworks we can avoid bias while working with a relatively small sample of human generated narratives, increasing efficiency and reducing the effort needed.
In this proposed approach we first compare the relatively smaller set of human narratives with the NLG narrative leveraging machine learning techniques for a first pass and then having the expert panel review a small subset of these comparisons.
An ideal machine learning technique will evaluate the narratives using different metrics. The first two metrics mentioned below focus on the “how” piece of the narratives while the third metric focuses on the “what” piece of the narratives.
The machine learning techniques are used to classify the results into three categories based on the accuracy:
We are entering an era where businesses and consumers will greatly benefit from the advances in the Natural Language Generation technologies. As we have further advances in AI, the technology will continue to become increasingly sophisticated and easier to scale and implement. Although an exciting time for NLG systems, the above-mentioned considerations and frameworks are critical to a successful selection of a NLG technology and a successful business scenario.